团队2018级研究生蒋光好同学的论文“An efficient attention module for 3d convolutional neural networks in action recognition”被SCI期刊《Applied Intelligence》 录用,祝贺!
Abstract:
Due to illumination changes, varying postures, and occlusion, accurately recognizing actions in videos is still a challenging task. A three-dimensional convolutional neural network (3D CNN), which can simultaneously extract spatio-temporal features from sequences, is one of the mainstream models for action recognition. However, most of the existing 3D CNN models ignore the importance of individual frames and spatial regions when recognizing actions. To address this problem, we propose an efficient attention module (EAM) that contains two sub-modules, that is, a spatial efficient attention module (EAM-S) and a temporal efficient attention module (EAM-T). Specifically, without dimensionality reduction, EAM-S concentrates on mining category-based correlation by local cross-channel interaction and assigns high weights to important image regions, while EAM-T estimates the importance score of different frames by cross-frame interaction between each frame and its neighbors. The proposed EAM module is lightweight yet effective, and it can be easily embedded into 3D CNN-based action recognition models. Extensive experiments on the challenging HMDB-51 and UCF-101 datasets showed that our proposed module achieves state-of-the-art performance and can significantly improve the recognition accuracy of 3D CNN-based action recognition methods.
Download: [官方链接] [preprint版本]
Keywords: Effective attention module, 3D CNN, Deep learning, Action recognition.
Photos:
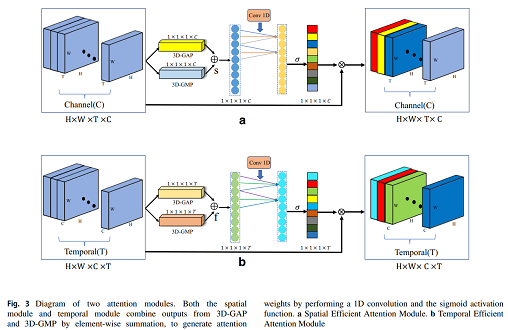
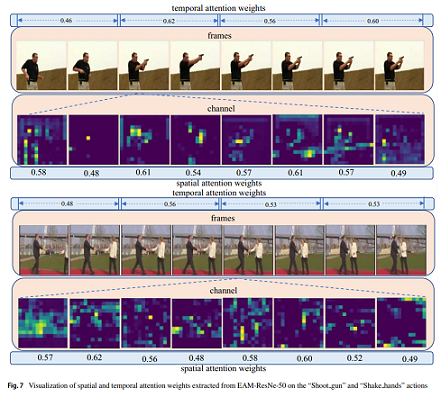