团队论文“PTQ4RIS: Post-Training Quantization for Referring Image Segmentation”被2025IEEE国际机器人与自动化会议《IEEE International Conference on Robotics and Automation》 接收,祝贺!
Abstract:
Referring Image Segmentation (RIS), aims to segment the object referred by a given sentence in an image by understanding both visual and linguistic information. However, existing RIS methods tend to explore top-performance models, disregarding considerations for practical applications on resources-limited edge devices. This oversight poses a significant challenge for on-device RIS inference. To this end, we propose an effective and efficient post-training quantization framework termed PTQ4RIS. Specifically, we first conduct an in-depth analysis of the root causes of performance degradation in RIS model quantization and propose dual-region quantization (DRQ) and reorder-based outlier-retained quantization (RORQ) to address the quantization difficulties in visual and text encoders. Extensive experiments on three benchmarks with different bits settings (from 8 to 4 bits) demonstrates its superior performance. Importantly, we are the first PTQ method specifically designed for the RIS task, highlighting the feasibility of PTQ in RIS applications.
Download: [preprint版本]
Keywords: PTQ,Referring Image Segmentation
Photos:
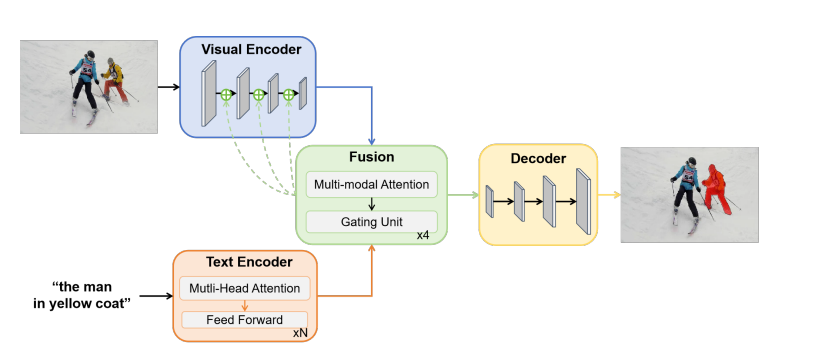