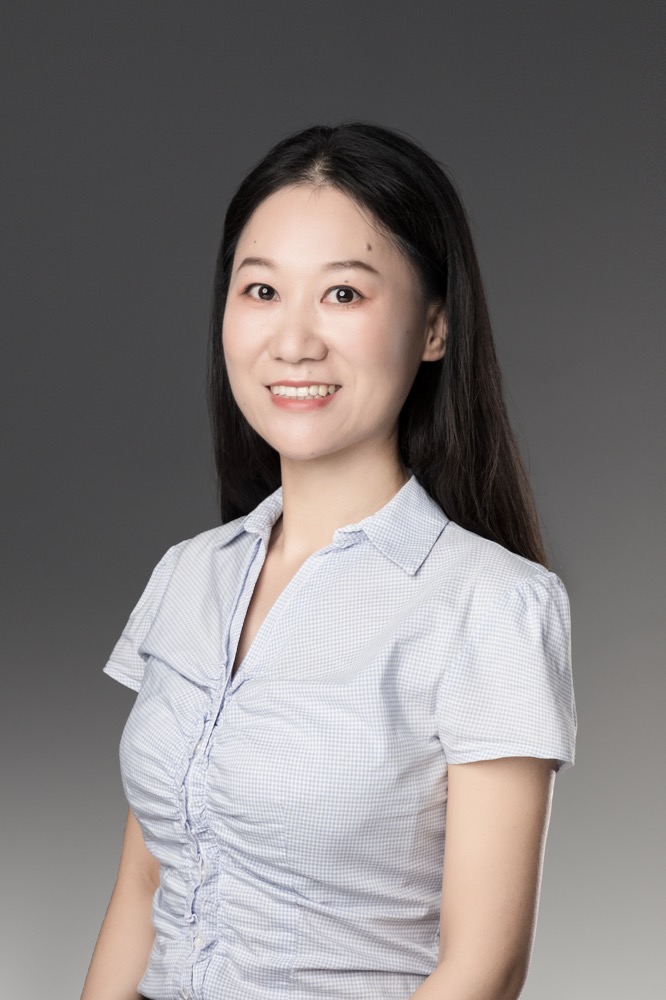
姜晓燕,副教授、硕导,博士毕业于耶拿大学(德国)计算机科学专业。
Xiaoyan Jiang is an Associate Professor at Shanghai University of Engineering Science since 2020.01. She is a Visiting Scholar in Leiden Institute of Advanced Computer Science (LIACS), Leiden University, Leiden, Netherlands. She got her doctor's degree in computer science from Friedrich-Schiller University Jena, Germany in 2015. She has published 60 publications in the field of computer vision and artificial intelligence. She serves as the Associate Editior of Applied Intelligence (IF: 3.9) since 2024.
讲授课程 Courses:计算机视觉 Computer Vision、机器学习 Machine Learning、数字图像处理 Digital Image Processing、面向对象程序设计 C++
链接 Links:谷歌学术 Google Scholar GitHub网页 荷兰莱顿大学访问学者页面 Homepage Leiden University
邮箱 E-mail:xiaoyan.jiang@sues.edu.cn
研究课题为计算机视觉、深度学习,应用领域包括视频监控、医疗辅助分析、工业检测、场景理解、智能交通等。在计算机视觉、人工智能领域发表论文60余篇,其中SCI/EI 50余篇,包括Trans. SMC, Trans. ITS, Pattern Recognition, Knowledge-Based Systems (KBS), SPIC, ICIP, ICONIP, ICME等。为多个顶级国际会议与期刊的评审。ICPCSEE2019、IEA/AIE2023的项目委员会成员、在CiSE2023,IWITC2021,ICFTIC2019国际会议做主旨报告。担任Applied Intelligence期刊副主编一职。曾获德国DAAD、中国政府奖学金CSC资助。学校青年五四奖章集体成员、上海市长宁区第四轮创新团队–智能视觉感知与信息处理创新团队核心成员、学院优秀教师。主持/参与国家自然科学基金青年项目、民航重点、面上、上海市教委项目、上海市科委重点项目、上海飞机制造有限公司项目等多项。申请发明专利八项,实用新型专利五项、软著多项。
现为电子与电气工程学院多维度人工智能科研团队负责人,团队注重科技研发的同时,积极推进人工智能技术的产业化。与各行业企业开展产学研合作,以5G + AI为未来模式,已在智能交通、三维场景建模、视频监控、瑕疵巡检、智慧医疗等方面取得多项成果,并应用到实际场景中。研究涵盖计算机视觉领域的多个课题:多目标跟踪、域自适应行人重识别、语义分割、视觉SLAM。所负责的项目应用到的领域:智能交通、视频监控、大型客机表面喷漆瑕疵检测、工业缺陷检测、胃癌淋巴结转移检测、眼震疾病诊断、心脏周期分析等。
团队以学生发展为中心,打牢从传统视觉算法到深度学习及大模型相关的关键知识与理论,结合实际场景,培养学生独立思考,发现问题和解决问题的能力。目标为激发大家持续终身学习的内驱力,最终团队得到成长和发展!如果你对自我有要求,对科学好奇,愿意为解决问题而努力,那本团队适合你,欢迎加入!
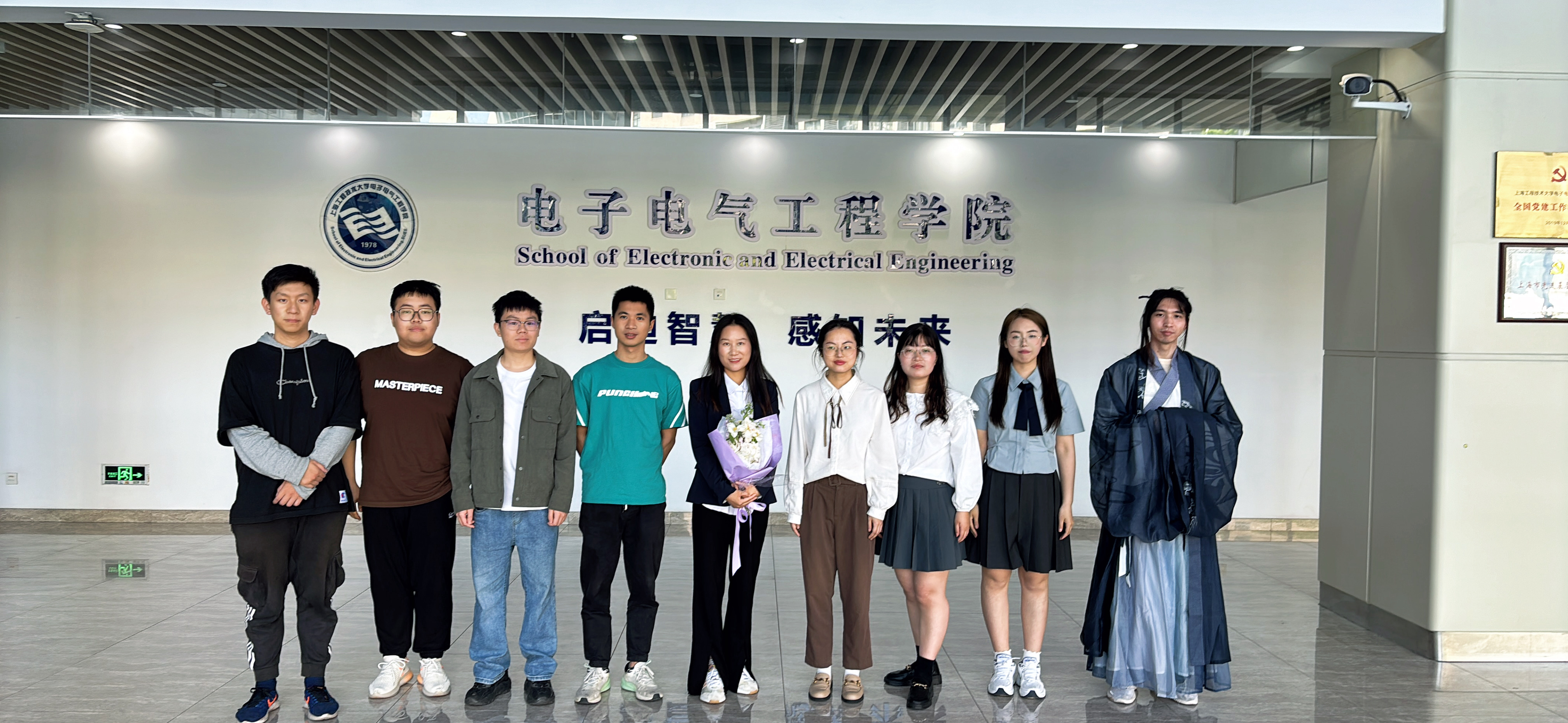