团队2018级研究生吴益同学的论文“Multi-modal 3D object detection by 2D-guided precision anchor proposal and multi-layer fusion”被SCI期刊《Applied Soft Computing》 录用,祝贺!
Abstract:
3D object detection, of which the goal is to obtain the 3D spatial structure information of the object, is a challenging topic in many visual perception systems, e.g., autonomous driving, augmented reality, and robot navigation. Most existing region proposal network (RPN) based 3D object detection methods generate anchors in the whole 3D searching space without using semantic information, which leads to the problem of inappropriate anchor size generation. To tackle the issue, we propose a 2D-guided precision anchor generation network (PAG-Net). Specifically speaking, we utilize a mature 2D detector to get 2D bounding boxes and category labels of objects as prior information. Then the 2D bounding boxes are projected into 3D frustum space for more precise and category-adaptive 3D anchors. Furthermore, current feature combination methods are early fusion, late fusion, and deep fusion, which only fuse features from high convolutional layers and ignore the data missing problem of point clouds. To obtain more efficient fusion of RGB images and point clouds features, we propose a multi-layer fusion model, which conducts nonlinear and iterative combinations of features from multiple convolutional layers and merges the global and local features effectively. We encode point cloud with the bird’s eye view (BEV) representation to solve the irregularity of point cloud. Experimental results show that our proposed approach improves the baseline by a large margin and outperforms most of the state-of-the-art methods on the KITTI object detection benchmark.
Download: [官方链接] [preprint版本]
Keywords: 3D object detection, Multi-modal, Autonomous driving, Feature fusion, Point cloud.
Photos:
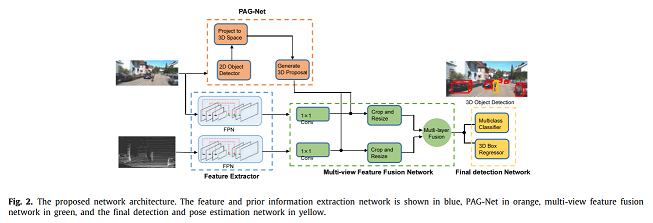
团队2018级研究生蒋光好同学的论文“An efficient attention module for 3d convolutional neural networks in action recognition”被SCI期刊《Applied Intelligence》 录用,祝贺!
Abstract:
Due to illumination changes, varying postures, and occlusion, accurately recognizing actions in videos is still a challenging task. A three-dimensional convolutional neural network (3D CNN), which can simultaneously extract spatio-temporal features from sequences, is one of the mainstream models for action recognition. However, most of the existing 3D CNN models ignore the importance of individual frames and spatial regions when recognizing actions. To address this problem, we propose an efficient attention module (EAM) that contains two sub-modules, that is, a spatial efficient attention module (EAM-S) and a temporal efficient attention module (EAM-T). Specifically, without dimensionality reduction, EAM-S concentrates on mining category-based correlation by local cross-channel interaction and assigns high weights to important image regions, while EAM-T estimates the importance score of different frames by cross-frame interaction between each frame and its neighbors. The proposed EAM module is lightweight yet effective, and it can be easily embedded into 3D CNN-based action recognition models. Extensive experiments on the challenging HMDB-51 and UCF-101 datasets showed that our proposed module achieves state-of-the-art performance and can significantly improve the recognition accuracy of 3D CNN-based action recognition methods.
Download: [官方链接] [preprint版本]
Keywords: Effective attention module, 3D CNN, Deep learning, Action recognition.
Photos:
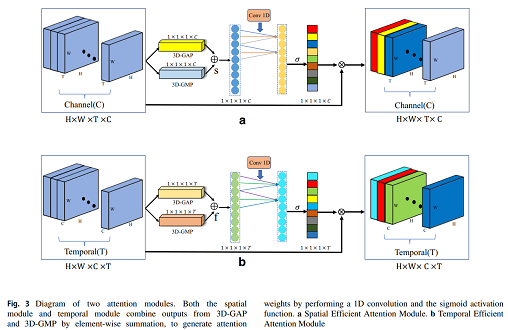
团队2018级研究生朱凯赢同学的论文“Photometric transfer for direct visual odometry”被SCI期刊《Knowledge-Based Systems》 录用,祝贺!
Abstract:
Due to efficient photometric information utilization, direct visual odometry (DVO) is getting widely used to estimate the ego-motion of moving cameras as well as map the environment from videos simultaneously, especially in challenging weak-texture scenarios. However, DVO suffers from brightness discrepancies since it directly utilizes intensity patterns of pixels to register frames for camera pose estimation. Most existing brightness transfer methods build a fixed transfer function which is inappropriate for successive and inconsistent brightness changes in practice. To overcome this problem, we propose a Photometric Transfer Net (PTNet) which is trained to pixel-wisely remove brightness discrepancies between two frames without ruining the context information. Photometric consistency in DVO is obtained by adjusting the source frame according to the reference frame. Since no dataset is available for training the photometric transfer model, we augment the EuRoC dataset by generating a certain number of frames with different brightness levels for each original frame by a nonlinear transformation. Afterwards, required training data containing various brightness changes and scene movements along with ground truth can be collected from the extended sequences. Evaluations on both real-world and synthetic datasets demonstrate the effectiveness of the proposed model. Assessment on an unseen dataset with fixed model parameters trained on another dataset proves the generalization ability of the model. Furthermore, we embed the model into DVO to preprocess input data with brightness discrepancies. Experimental results show that PTNet-based DVO achieves more robust initialization and accurate pose estimation than the original one.
Download: [官方链接] [preprint版本]
Keywords: Photometric transfer, Direct visual odometry, Data augmentation, Brightness discrepancy, Deep learning.
Photos:
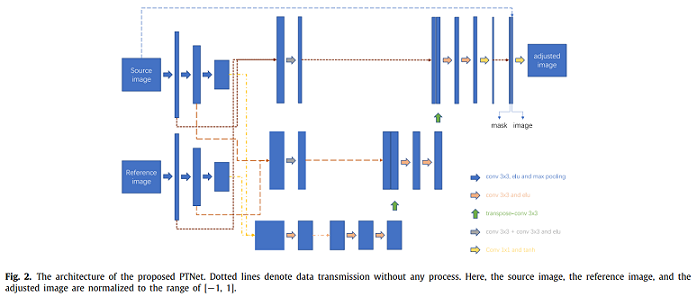