团队2017级研究生黎阳同学的论文“Effective Person Re-identification by Self-Attention Model Guided Feature Learning”被SCI期刊《Knowledge-Based Systems》 录用,祝贺!
Abstract:
Person re-identification (re-ID), of which the goal is to recognize person identities of images captured by non-overlapping cameras, is a challenging topic in computer vision. Most existing person re-ID methods conduct directly on detected objects, which ignore the space misalignment caused by detectors, human pose variation, and occlusion problems. To tackle the above mentioned difficulties, we propose a self-attention model guided deep convolutional neural network(DCNN) to learn robust features from image shots. Kernels of the self-attention model evaluate weights for the importance of different person regions. To solve the local feature dependence problem of feature extraction, the non-local feature map generated by the self-attention model is fused with the original feature map generated from the resnet-50. Furthermore, the loss function considers both the cross-entropy loss and the triplet loss in the training process, which enables the network to capture common characteristics within the same individuals and significant differences between distinct persons. Extensive experiments and comparative evaluations show that our proposed strategy outperforms most of the state-of-the-art methods on standard datasets: Market-1501, DukeMTMC-reID, and CUHK03.
Download: [官方链接] [preprint版本]
Keywords: Personre-identification, Feature extraction, Self-attention, Cross-entropy loss, Triplet loss.
Photos:
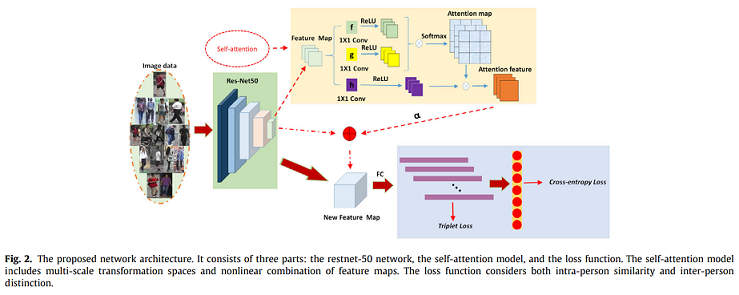
2019年9月21日,应AEIC组委会邀请,团队负责人姜晓燕博士前往大连参加International Conference on Frontiers Technology of Information and Computer会议,并做主旨报告。
团队负责人姜晓燕老师的论文“Unsupervised learning of depth and ego-motion with spatial-temporal geometric constraints”在IEEE International Conference on Multimedia and Expo (ICME) 中被接收,祝贺!
Abstract:
In this paper, we propose an unsupervised joint deep learning pipeline for depth and ego-motion estimation that explicitly incorporated with traditional spatial-temporal geometric constraints. The stereo reconstruction error provides the spatial geometric constraint to estimate the absolute scale depth. Meanwhile, the depth map with absolute scale and a pre-trained pose network serve as a good starting point for direct visual odometry (DVO), resulting in a fine-grained ego-motion estimation with the additional back-propagation signals provided to the depth estimation network. The proposed joint training pipeline enables an iterative coupling optimization process for accurate depth and precise ego-motion estimation. The experimental results show the state-of-the-art performance for monocular depth and ego-motion estimation on the KITTI dataset and a great generalization ability of the proposed approach.
Download: [官方链接]
Keywords: Depth, ego-motion, geometric constraint, visual odometry
Photos:
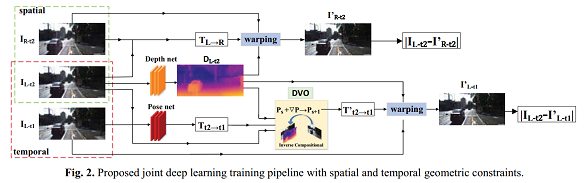
团队负责人姜晓燕老师的论文“An End-to-End Human Segmentation by Region Proposed Fully Convolutional Network” 被SCI期刊IEEE Access接收,祝贺!
Abstract:
Person segmentation in images has various applications, for example, smart home, human-computer interaction, and scene perception for self-driving cars, which are a key feature of the Internet of Things. Due to limitations in performance, such as accuracy and runtime, most traditional methods do not fulfill the practical requirements. Deep learning-based modern segmentation systems become prevalent. Fully convolutional network (FCN), as a classic image semantic segmentation method, directly optimizes the semantic map from the original image in a pixel-wise manner without using pixel-correlations or global object information. In this paper, we propose an efficient end-to-end person segmentation network structure fusing the person detection network with the FCN. The person detection network estimates the region of interest of persons and enforces the segmentation network to focus on the optimization of person segmentation. The loss function of the proposed network considers both the segmentation error and the detection bias error. In addition, the lightweight design of the detection network that optimizes only person bounding-box coordinates enables real-time person detection. The experimental comparison and analysis of several different networks on several datasets show the effectiveness of the proposed fusion strategy. The approach shows a promising practical application potential by fast running time and high segmentation accuracy.
Download: [官方链接]
Keywords: Person segmentation, fully convolutional network, object detection network, internet of things.
Photos:
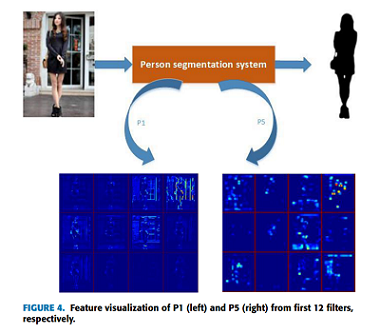