团队负责人姜晓燕老师的论文“Data Fusion-Based Multi-Object Tracking for Unconstrained Visual Sensor Networks” 被SCI期刊IEEE Access接收,祝贺!
Abstract:
Camera node perception capability is one of the crucial issues for visual sensor networks, which belongs to the field of Internet of Things. Multi-object tracking is an important feature in analyzing object trajectories across multiple cameras, thus allowing synthesis of data and security analysis of images in various scenarios. Despite intensive research in the last decades, it remains challenging for tracking systems to perform in real-world situations. We therefore focus on key issues of multi-object state estimation for unconstrained multi-camera systems, e.g., data fusion of multiple sensors and data association. Unlike previous work that rely on camera network topology inference, we construct a graph from 2-D observations of all camera pairs without any assumption of network configuration. We apply the shortest path algorithm to the graph to find fused 3-D observation groups. Our approach is thus able to reject false positive reconstructions automatically, and also minimize computational complexity to guarantee feasible data fusion. Particle filters are applied as the 3-D tracker to form tracklets that integrate local features. These tracklets are modeled by a graph and linked into full tracks incorporating global spatial-temporal features. Experiments on the real-world PETS2009 S2/L1 sequence show the accuracy of our approach. Analyses of the different components of our approach provide meaningful insights for object tracking using multiple cameras. Evidence is provided for selecting the best view for a visual sensor network.
Download: [官方链接]
Keywords: Data fusion, graph theory, Internet of Things, particle filters, visual sensor networks.
Photos:
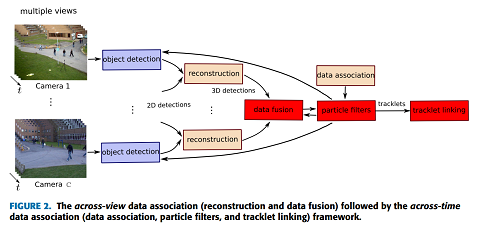